Using Large Language Models and AI Agent Systems to Provide AI Assistants for Improved Industrial Operations
The new wave of AI technology is fundamentally changing how we think about interacting with data. Natural language interfaces in tandem with AI agent systems are presenting multiple opportunities to increase productivity, efficiency and innovation across an ever increasing range of everyday tasks. General purpose tools like ChatGPT, Copilot, Gemini, Claude and Perplexity are becoming more ubiquitous in our daily lives. Substantial investments and soaring valuations of companies like OpenAI and Anthropic show that this revolution is only just beginning. As with cloud computing before it, the role of AI assistants which learn and improve over time will rapidly move from a curiosity to becoming a core pillar of organizational digital transformation strategies; we are moving from the era of democratized data to that of democratized insights.
Already the integration of large language model (LLM) based text and voice assistants into the wider enterprise software ecosystem is rapidly accelerating.
At Arundo we spent much of 2024 asking ourselves what this paradigm shift will mean for heavy-asset and process-intensive industries in 2025 and beyond. We have worked with our current customers in applying these state of the art AI approaches to their systems and process data and as a result have made several core observations that are now guiding our strategy in bringing this offering to GA release in the coming months. In particular:
- AI assistants will minimize the time to insight from data and maximize the number of actionable insights available for decision makers within operations teams.
- AI agents, when paired with configurable domain graph models and scalable advanced analytics, can provide high-value insights from industrial data including telemetric sensor data.
- Team collaboration within and across AI assistant conversations needs to be front and center to enable efficient data-driven decision making in operations.
- AI assistant conversations must be accessible and transferable across devices and platforms to meet users where they are.
- Concerns around trust and the transparency of AI assistant responses can be alleviated through a combination of robust architecture and clearly designed user interfaces.
Boosting Productivity in Industrial Operations through a Ready-Made and Versatile AI Agent Architecture
Human-in-the-loop AI assistants can reduce the burden on digital teams by providing decision-makers with a wide variety of on-demand insights derived directly from data. This eliminates the need for extensive consultation with analysts and subject matter experts. It also reduces dependence on those who update and maintain fixed data dashboards or generate custom reports for operations teams. The flexibility of AI agent-based workflows allows for responses that include graphical and tabular output. This provides operations teams with the ability to generate dashboards and reports using their own fine-tuned natural language prompts as their needs evolve. Additionally, AI assistants can schedule the running of such prompts, offering a compelling and flexible alternative to traditional dashboarding and reporting tools.
In heavy-asset operations, the majority of required insights rely on accurately understanding the current state of complex, dynamic systems and processes. Therefore, an AI assistant must possess the capability to efficiently pinpoint relevant information, retrieve necessary data, and generate clear, actionable responses to highly domain-specific queries. The ability to extract key information from sensor time-series data is of particular importance. To achieve this, the underlying technical architecture must effectively combine the strengths of LLMs with an interpretive domain knowledge layer, with access to both metadata and relevant sensor readings for processes and systems. The architecture must also be modular, allowing for the
adoption of new innovations in AI technologies as they become available. We discuss elsewhere how the combination of knowledge graphs, computes and LLM based AI agents provides a powerful foundation for such an architecture.
Using AI Assistants for Collaboration between Industrial Operations Teams and Departments
The dynamic nature of heavy-asset and process-intensive industries requires more than just data-driven insights being available on demand; it requires close coordination between different teams and departments. To facilitate effective decision-making, interactions with an AI assistant must therefore be accessible to all relevant stakeholders. Moreover, to maintain accountability, the information used to inform critical decisions must be auditable. Therefore, an AI assistant should enable multiple stakeholders to participate in and review ongoing conversations. The assistant conversations should be easily retrievable and strive for reproducibility whereby running the same conversation on identical input data should yield consistent results. By prioritizing collaborative workflows the AI assistant becomes a core tool in making decisions efficiently and with confidence; it effectively becomes a virtual member of the decision making team.
Efficient collaboration requires that an AI assistant must be accessible and adaptable across platforms and devices. Not only should its functionality be available in a standalone web application, it should also meet users where they already collaborate. The more ubiquitous its presence, the more the insights provided by an AI assistant become an integral part of the team's toolkit. Whether team members are on the factory floor with a mobile device or already communicating using an existing productivity tool such as Teams, ready access to the AI assistant conversations across these platforms will prevent information retrieval becoming a bottleneck to efficient decision making.
Maintaining Trustworthyness and Transparency for AI Assistants in Industrial Operations
In process-intensive industries, the consequences of an incorrect insight can be as severe, if not more so, than the benefits of a correct one. Traditionally, this has led to a reliance on human-in-the-loop systems, where operators retain ultimate decision-making authority. While an AI assistant inherently maintains this human oversight, its architecture must also address potential "hallucinations" or inaccuracies in LLM-generated responses, which could erode trust. Although ongoing advancements in foundational LLM models are continually improving accuracy, domain-specific solutions can further enhance reliability through a deeper understanding of the specific context and careful prompt engineering. To foster user confidence, application interfaces should prioritize transparency by allowing users to define the level of constraint applied to assistant responses and directly inspect the underlying data sources and associated assumptions used to generate those responses. This approach promotes trust and empowers users to make informed decisions based on verifiable information.
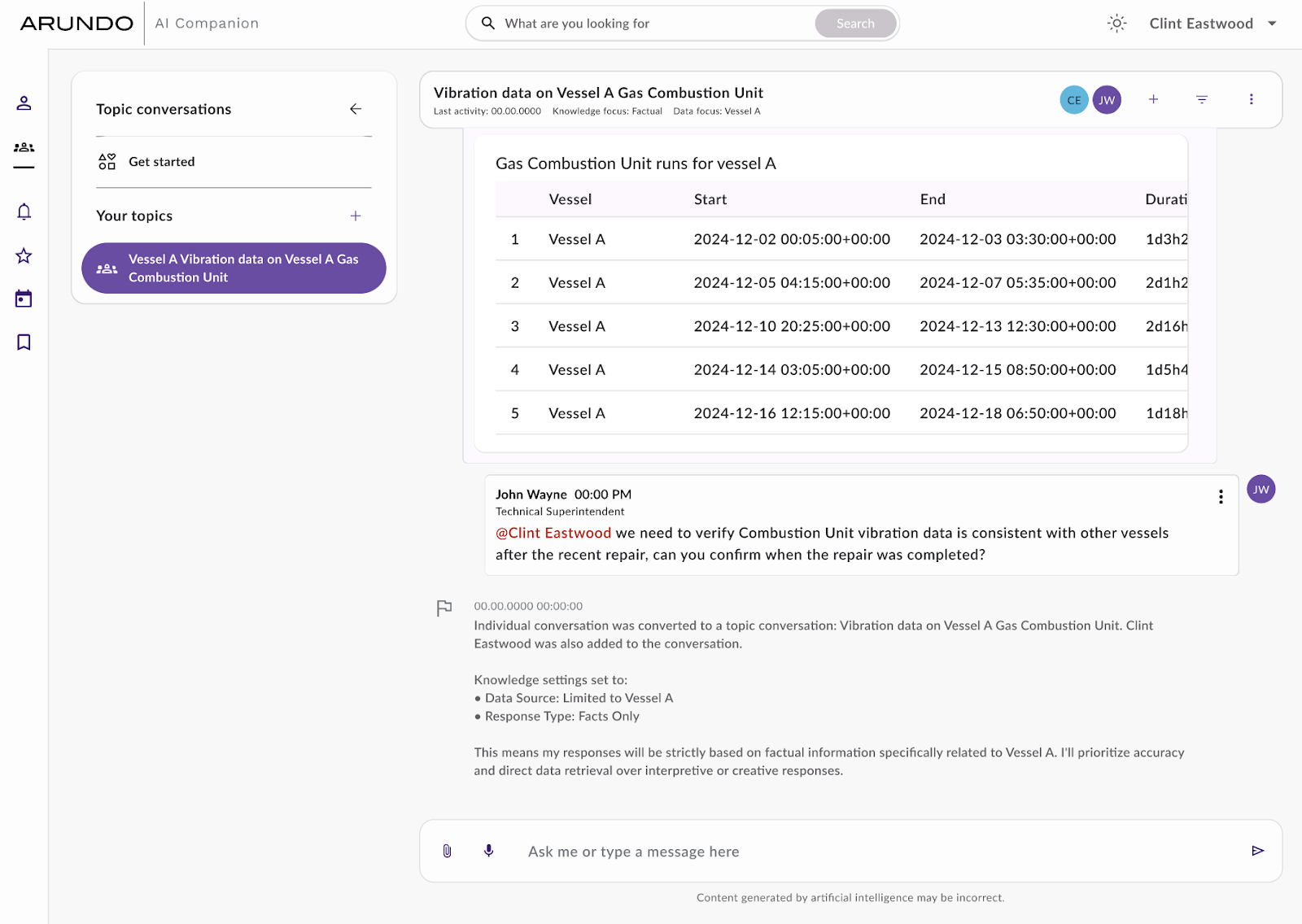
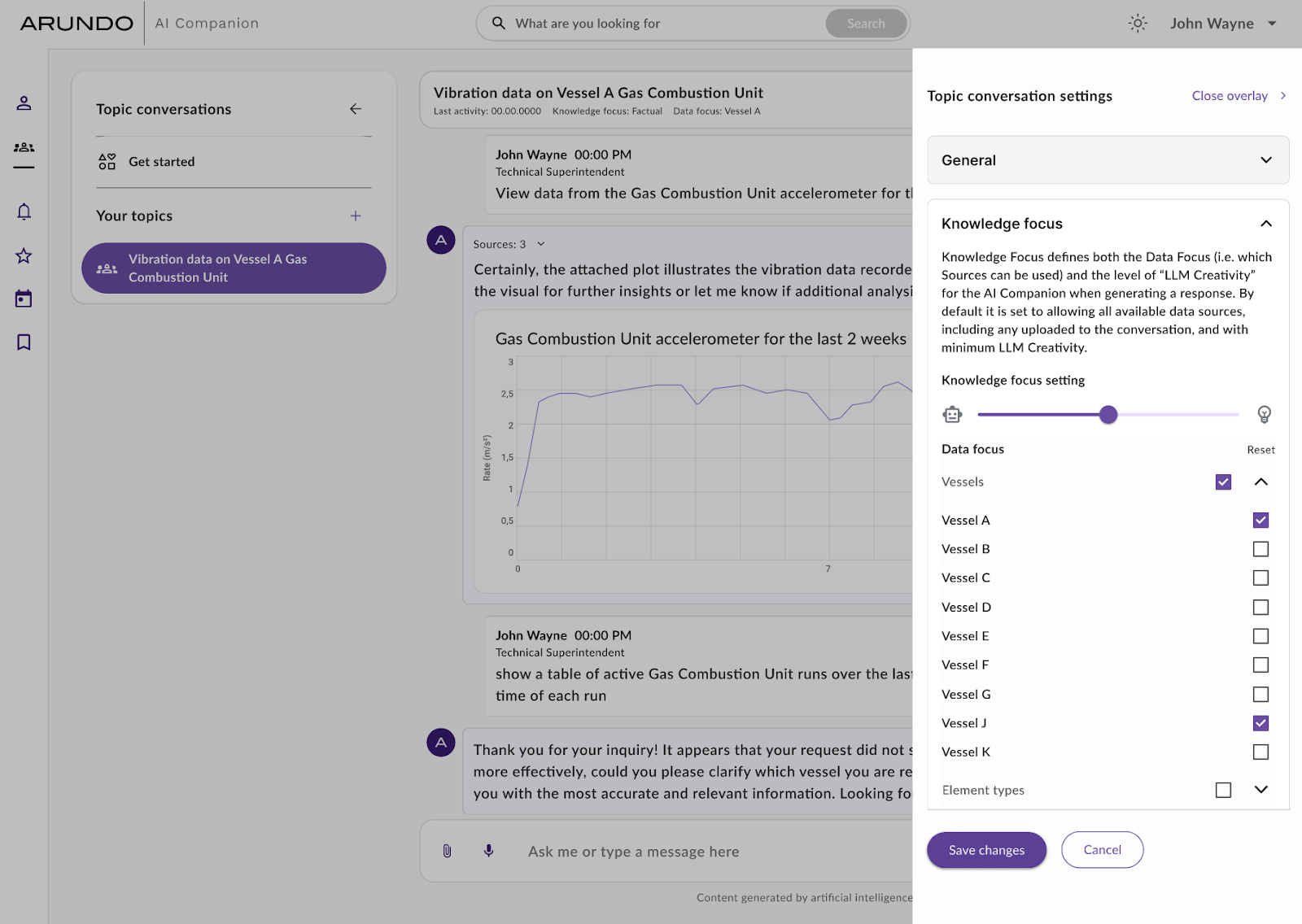
Getting Started with a Ready-Made AI Assistant Tailored for Industrial Operations
The successful integration of AI assistants to support more efficient decision-making in heavy-asset and process-intensive industries hinges on a multifaceted approach. It requires a thoughtful combination of flexible user-centric interfaces, focusing on team collaboration, adaptable architecture and a commitment to transparency and accuracy. At Arundo we believe that by embracing these principles, we can leverage our existing industry specific knowledge and technology experience so that organizations can unlock the full potential of AI assistants, fostering a new era of data-driven decision-making and operational excellence. We are excited with what we have already accomplished with our current customers and are keen to engage with additional organizations interested in joining us on this journey. Many tools are now providing organizations the ability to build their own AI agent systems. This is suitable for organizations with large digital teams and appropriate in-house expertise, but we are embracing the challenge of developing a ready-made robust and versatile AI agent architecture specifically for those companies who are not looking to build their own systems from the ground up. Please contact us if you would like to learn more.