It’s easy to get carried away with the hype around digital twins. However, what’s a digital twin? How can you get the most value out of your digital twin?
It’s easy to get carried away with the hype around digital twins. The term has been thrown around for a few years now and has also been in Gartner’s Top 10 Strategic Technology Trends in 2017, 2018 and 2019. However, what’s a digital twin? How can you get the most value out of your digital twin?
WHAT’S A DIGITAL TWIN?
The concept of the digital twin originated from Dr. Michael Grieves, Chief Scientist of Advanced Manufacturing at the Florida Institute of Technology in 2002 in Product Life Cycle (PLC) courses. He proposed the digital twin as the conceptual model underlying PLC Management. Since then there’s been some further development.
Simply put, a digital twin is a virtual model of your real-world, physical processes, product or service. The digital twin collects real-time data through sensors and combines this with analytics technology. Pairing this virtual model with your physical equipment or asset allows you to better understand, monitor, analyze, manipulate or optimize your assets and equipment. You’ll be able to uncover problems and opportunities in the virtual environment and apply them in the physical world. You’ll be able to run experiments on the digital twin first without risking unplanned downtime or disruption with the real equipment.
EXAMPLES OF DIGITAL TWINS
Modeling assets or equipment to monitor operational and maintenance performance isn’t new.
The digital twin moves beyond operational and maintenance capabilities due to the range of data sources applied to the model and the advanced capabilities it can accommodate. The more data it’s gathering, the more accurately it can model performance and potential outcomes. Likewise, the more data streams that are integrated the complexity multiplies.
Digital twins have grown in complexity and sophistication and can range from a digital representation from one single item to models of systems of interconnected things. They can be as simple or complicated as you’d like. Like many early technology trends, well-known challenges often overshadow small successes and that’s especially true with digital twins. The most successful users of digital twins often start with narrowly scoped focus areas and use cases before investing in platforms or large-scale representations of complex systems.
SIMPLE EXAMPLE
Consider a pump system. To enhance a traditional model of a pump system, you have to not only model and measure the physical components, such as the hydraulic system, electrical system, and controls.
You could also consider application-specific elements, such as fluid characteristics that may change and affect materials and performance of the system over time. You might also want to integrate specific historical events, such as time in service, part history, failure events, and maintenance records. You might want to include manufacturer specifications and performance expectations, known tolerance thresholds, and any other first principles information about how the pump system should work.
Ideally, a digital twin could integrate all of this information, along with machine learning and other advanced analytics, to improve your understanding of the system. But this is just the first step. This data integration is only helpful if it provides guidance to make better decisions, or reduces cost or complexity of managing the pump system.
A common point of failure for digital twin projects is that the efforts focus first on data integration, rather than business processes and value creation opportunities.
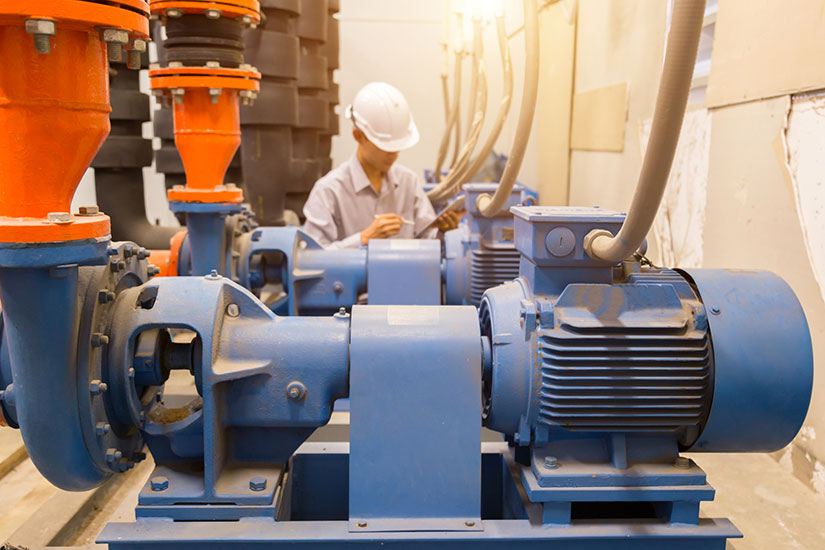
COMPLEX EXAMPLE
Rather than a single pump system, your users might get more value from modeling an entire process unit, or an entire plant or platform. This could involve multiple pump systems as well as other equipment, both rotating and static, and may also involve complex, sometimes proprietary, chemical or physical processes. Traditionally, understanding such complex systems starts with first principle-based simulations, which may dynamically update based on real-time data.
The primary advantage of a digital twin in such instances is to combine simulations with data science and machine learning models, in addition to advanced data integration that orchestrates interchange among a variety of existing information systems. Especially in highly bespoke and complex technological environments, the value of this digital twin is in understanding how a system operates, how it should operate, how it could operate better – and what you can do about this in a time frame that makes sense for your business.
WHERE SHOULD YOU START TO GET THE MOST VALUE?
In a study run by Gartner on organizations that had already implemented internet of things (IoT), 13% of the respondents already use digital twins and 62% are already planning or establishing digital twins within the next year. However, there’s no one approach to implementing digital twin technology that’s right for all companies or use cases. In fact, the definition and scope of digital twins vary widely among companies, even within the same industry.
In reality, digital twin initiatives that start by implementing a big vision may suffer from schedule and budgetary challenges. In our experience, the most successful digital adopters often start with specific narrow use cases and build digital capabilities horizontally.
For every digital initiative, we recommend that you focus on the business problem(s) you need solved and the business value you’re creating. Build an infrastructure around that, learn from your experiences and scale over time.
- What business problem(s) do you need solved?
- Where can you get the most value and what’s technically feasible to do given your current systems?
- Do you need to make infrastructure investments to test hypotheses around value and feasibility, or can you prototype solutions first to better understand future investments?