Today’s oil and gas production engineers face several challenges ensuring optimal well production. They're now looking at digital technologies and data science to solve these challenges.
Today’s oil and gas production engineers face several challenges to ensure that their wells are achieving optimal production. Companies are now looking at digital technologies and state-of-the-art data science to solve these challenges.
THE SHALE BOOM HAS TRANSFORMED AND REVOLUTIONIZED THE OIL AND GAS INDUSTRY
The U.S. shale boom over the last decade has transformed and revolutionized the oil and gas industry. The advancements in ‘fracking’ and ‘horizontal drilling’ technologies have allowed operators to extract oil and gas trapped in all major shale plays in the US, in a more economical way than in the past. Due to technological advances, these wells can be drilled and completed in less than 45 days. Because of these factors, there has been a tremendous increase in the number of active wells in North America and a commensurate increase in hydrocarbon production. According to US Energy Information Administration (EIA), for the first time since the 1950s, United States became a ‘net energy exporter’ in September 2019.
The net effect of this high volume, factory-like ‘commoditization’ of shale drilling and completions is that today’s production engineers have to consistently manage many more active wells than they have in the past, with the same or fewer employees. To make sure the wells are producing at their maximum potential at all times, production engineers often have to make a critical choice - to focus their attention on a small number of the most critical assets, while neglecting the rest. This, in turn, sometimes results in a well producing lower than its potential for a long time. Sometimes even months go by before they recognize the decrease in production and determine that the well requires some type of intervention.
Industry data reflects this operational reality. As few as 20% of existing wells are actively managed by production professionals, while as many as 80% go unmanaged and are therefore sub-optimized. We recently worked with a large operator in the Marcellus Shale who indicated that as many as 30% of all gas wells they operate can be non-producing on a single day. This non-productive time is driven by various operational issues such as failure of hydraulic power units, solids eroding well necks, liquid loading of production tubing, sand covering casing perforations, etc. Additionally, due to the dynamically changing well profiles of the unconventional wells, managing and optimizing them requires near real-time, high-frequency data, as well as quick turnarounds on the solutions when a problem is encountered with production.
CHALLENGES OF SUB-OPTIMIZED PRODUCTION
The problem of providing near real-time well telemetry data (pressures, temperatures and flow rates) has had the undivided attention from many industry players in the last decade. In fact, the widespread focus on this has now led to an overwhelming amount of information being available to remote operators and engineers at their fingertips, and at a fraction of the cost due to cheaper, more reliable networks. As a result, the industry focus has now shifted from ‘getting access to high-frequency data in near real-time’ to ‘how can I filter the noise and focus on the most critical data?’
These challenges can be summarized into following tactical tasks you can focus on:
- How can I quickly identify critical assets with unanticipated declines in production - the "Biggest Losers"?
- How do I optimize the trade-offs between the accuracy of the solution for solving the problem on one well versus scaling a ‘good enough’ solution to hundreds of wells?
- How can I reduce alarm fatigue caused by false alarms?
DIGITAL TECHNOLOGIES TO THE RESCUE
Digital technologies like the industrial internet of things (IIoT), machine learning, artificial intelligence (AI), cloud, and edge computing are now uniquely positioned to solve some of these critical challenges. They can help you optimize production by providing you with accurate, actionable and timely insights to make better decisions for optimizing the overall hydrocarbon production.
Using state-of-the-art data science techniques, you can develop machine learning models that utilize the well telemetry data to detect sudden and unanticipated deviations in the well pressure profiles. Further, you can also use various modeling approaches to forecast future production rates based on recent trends. Alarms can then be set on various data points as they deviate from the forecasted values, or if there’s higher than expected deviation from the recent trend to alert the human-in-the-loop operator. Due to the dynamic nature of the unconventional shale wells, it’s also critical that you experiment with various window sizes of historical data to find the optimum window size to make predictions and detect deviations.
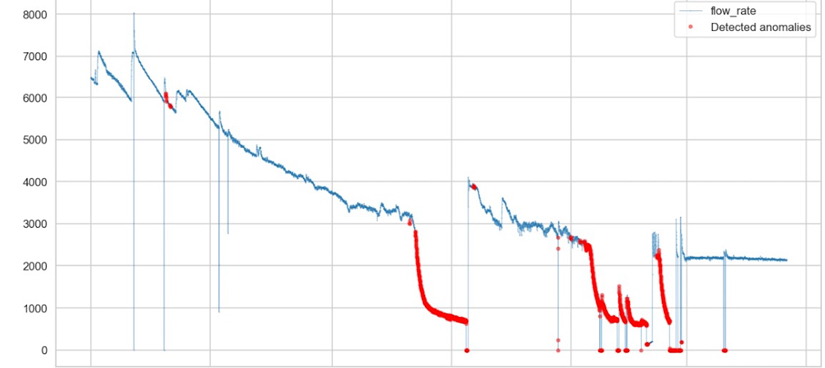

Just like most solutions, there's no ‘silver bullet’ model that solves this all. In our recent pilot work with a large independent natural gas producer in the Marcellus Shale, we found that a ‘hybrid’ approach involving 2 different modeling techniques worked best. This best-of-both-worlds’ approach gave the optimum trade-off between the accuracy of detecting deviations in the well pressure profiles, and the ease of scalability across hundreds of wells. By weeding out false alarms, and providing fairly accurate predictions and trends, these AI technologies enable production engineers to always focus their attention on the ‘Biggest Losers’.
ABOUT THE AUTHORS
Divye Kumar worked as a Product Manager at Arundo Analytics' Houston office. He led Arundo's various product discovery and development initiatives in the Upstream Oil & Gas sector.
At Arundo, Piers Wells led sales in the Americas and Arundo's global technology partnerships. He is an experienced digital technology entrepreneur with over 20 years of experience in the energy and broader industrial sectors.